Outstanding Info About How To Avoid Overfitting
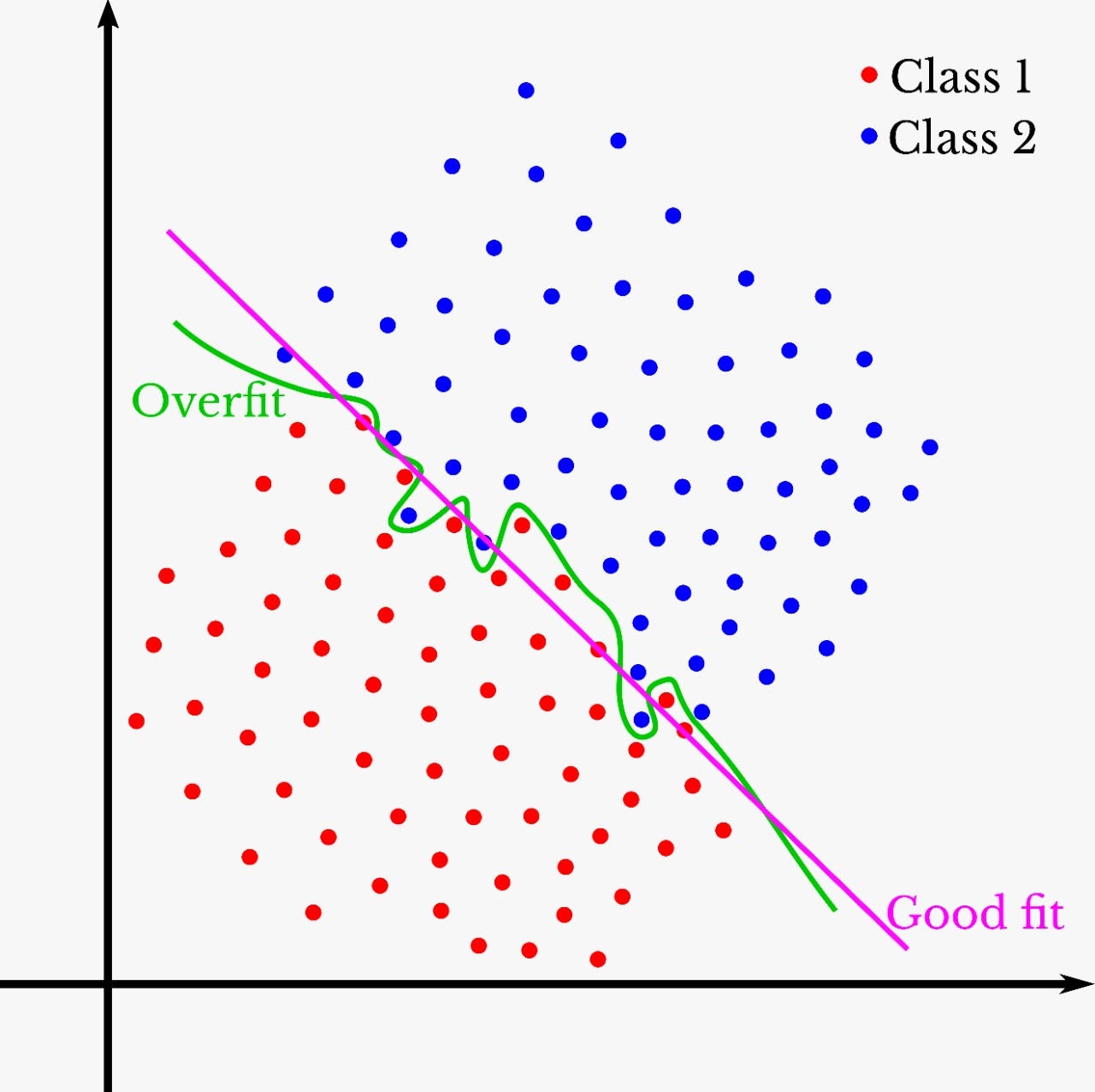
Train with more data — training with more data can help the model determine trends in the data in order to make more accurate predictions.
How to avoid overfitting. To avoid overfitting, just change the learning set on each analysis. Having detected overfitting, now the model needs to be rid of it. There are various ways in which overfitting can be prevented.
However, the reality is you are probably not starting a strategy at the beginning. Overfitting is an undesirable machine learning behavior that occurs when the machine learning model gives accurate predictions for training data but not for new data. Diversity of data and a larger dataset is the easiest way to avoid overfitting of the model.
This is well known fact. In the most egregious cases, an overfitted model assumes that the feature value combinations seen during training will always result in the exact same. The model attempts to capture the data points that do not represent the accurate properties of data.
I’ll start with the most straightforward method you can employ. Overfitting impacts the accuracy of machine learning models. Increasing the volume of your data in the training.
I’m going to start off with the simplest technique you can use. There are two ways to approach an overfit model: Pruning refers to a technique to remove the parts of the decision tree to prevent growing to its full depth.
Running a backtest during bull and bear markets is a good first step. Reduce overfitting by training the network on more examples. The simplest way to reduce overfitting is to increase the size of the training data.